Don’t believe the damned lies about statistics
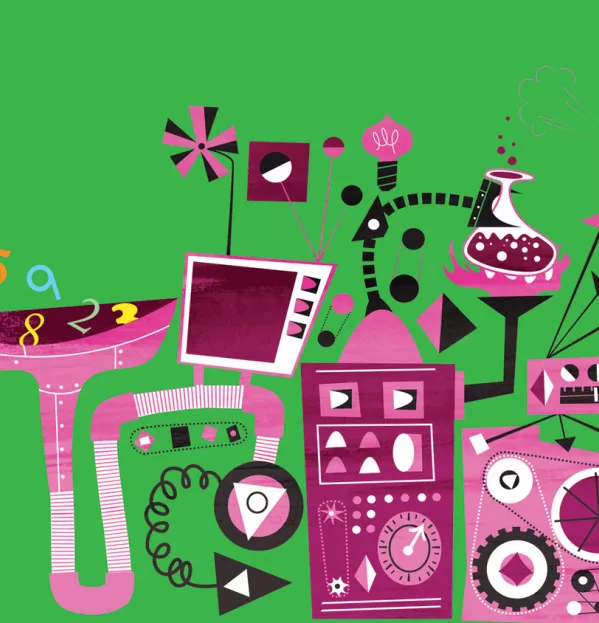
I believe in statistics. I am convinced that careful presentation of data - and, yes, a little bit of mathematics - can help us to better understand our students.
So I think that it’s well worth school leaders making the effort to ensure that everyone in a school learns the concepts associated with proper statistical presentation. But that’s not so easy.
Good data and bad data
The chief lesson of statistics is that even the best numbers will involve uncertainty, error and noise, perhaps from many different sources.
One of the first problems that a course in statistics teaches you to resolve is this: how different is different? Or, in other words, is variation in data due to uncertainty? Or is it due to something interesting?
The catacombs of statistical science are lined with bad ideas about how to answer that question. The typical method works something like this: first, you assume a baseline level against which to compare. You might take the scores on a literacy test at the beginning of the year, or mile-run times at the start of an exercise programme.
At GCSE or A level, a usual comparison is against a theoretical average school. (Every school wants to know whether it’s doing better than most of the others.)
The most common mistake is to assume that this school would always get average results - ie, results that are better than about half of other schools and worse than the remaining half. Just think of the diversity of students and intakes that most schools have. Even a school that performs in an average way would have these variations, which can sometimes be substantial. The theoretical average school sometimes would appear high in the league tables, and sometimes it would appear low.
The question “Are we better than average?” becomes “Are we doing well enough that we can be sure we would nearly always beat the theoretical average school, despite its natural variation?”
That’s the key question, and it stands on a teetering Jenga tower of assumptions.
Take the question of the relevant “average” school, for example. If we take the simple mean of national results, we must include lots of schools in less challenging social conditions than yours. Schools in different regions. Selective, independent or local authority schools. All of these factors could skew the average and either advantage or disadvantage your school.
Picking the right model
The promise of statistics is that they help us to evaluate specific questions objectively. Which questions to ask, however, is a question on which statistical science is agnostic. Multiple kinds of models with different limitations exist to answer different questions. When it seems that there is a haze of conflicting statistics, the reason is that the data user has not properly decided what they want out of their numbers.
Typical scientific articles are mostly made up of arguments as to why the chosen statistical procedures are important and necessary. The actual data, by contrast, flies by.
In schools, we need to learn from this. Without discussion and understanding of the purpose and flaws of our analyses, especially value-added ones, they are likely to be misleading or misinterpreted. Used carefully, data analysis is all the more meaningful.
But using statistics conscientiously is hard. Even if you get everything right, when do you decide that the evidence is strong enough to definitively show that your school is amazing? Here, again, it is less than easy to be rigorous. Typically, data providers (eg, ALIS) suggest a threshold above which it’s safe to say that your school has exceptionally good results.
‘If used carefully, data analysis is all the more meaningful’
That’s because above the cut-off the probability is low (conventionally, less than one in 20) that the national average school, with results adjusted for the background of your students, would have produced results like yours.
It’s unlikely that a school truly performing at the average level, in other words, could have achieved at your level - so you must be above average.
That one in 20 figure should be up for suspicion. Beating only 18 out of 20 hypothetical schools is still pretty good and probably would merit inclusion in the annals of excellent schools. How about 17, then? The need to choose a cut-off starts to feel a bit shaky.
And how about the opposite question: it looks like the chemistry department has beaten the cut-off - does that mean that the chemistry department is definitely doing something right?
No. It’s a little embarrassing, but I’m afraid that’s the answer. If the chemistry department isn’t very big, or if there are many departments in the school or sometimes for no reason at all, the chemistry department may have just gotten lucky.
Light out of the darkness
With all of the uncertainty in statistical methods, many people feel that they can’t evaluate statistical claims. “Oh, it’s just statistics, it can say anything,” or “I know that the stats say X, but I know Y” are two of the more common thoughts that non-specialists have.
I think that these ideas are unhelpful. We need to recognise that data is incredibly powerful because it is less subjective.
Personal judgements are inevitably swayed by unconscious biases, but the data never make excuses for its friends or gets irritable because it is hungry.
For that objectivity, we trade clarity. Every new use of data must come with a nuanced understanding of the implicit assumptions of the use of the data.
Discussing and analysing these ideas allows for more precise analyses that cut through the fog of numbers. This, in turn, lets us ask better questions, get better answers and, ultimately, get better outcomes for our students. That’s what counts.
Mark Chonofsky is a maths teacher in London
You need a Tes subscription to read this article
Subscribe now to read this article and get other subscriber-only content:
- Unlimited access to all Tes magazine content
- Exclusive subscriber-only stories
- Award-winning email newsletters
Already a subscriber? Log in
You need a subscription to read this article
Subscribe now to read this article and get other subscriber-only content, including:
- Unlimited access to all Tes magazine content
- Exclusive subscriber-only stories
- Award-winning email newsletters